Expo
view channel
view channel
view channel
view channel
view channel
view channel
view channel
RadiographyMRIUltrasoundNuclear MedicineGeneral/Advanced Imaging
Industry News
Events
- AI Improves Early Detection of Interval Breast Cancers
- World's Largest Class Single Crystal Diamond Radiation Detector Opens New Possibilities for Diagnostic Imaging
- AI-Powered Imaging Technique Shows Promise in Evaluating Patients for PCI
- Higher Chest X-Ray Usage Catches Lung Cancer Earlier and Improves Survival
- AI-Powered Mammograms Predict Cardiovascular Risk
- Cutting-Edge MRI Technology to Revolutionize Diagnosis of Common Heart Problem
- New MRI Technique Reveals True Heart Age to Prevent Attacks and Strokes
- AI Tool Predicts Relapse of Pediatric Brain Cancer from Brain MRI Scans
- AI Tool Tracks Effectiveness of Multiple Sclerosis Treatments Using Brain MRI Scans
- Ultra-Powerful MRI Scans Enable Life-Changing Surgery in Treatment-Resistant Epileptic Patients
- Novel PET Imaging Approach Offers Never-Before-Seen View of Neuroinflammation
- Novel Radiotracer Identifies Biomarker for Triple-Negative Breast Cancer
- Innovative PET Imaging Technique to Help Diagnose Neurodegeneration
- New Molecular Imaging Test to Improve Lung Cancer Diagnosis
- Novel PET Technique Visualizes Spinal Cord Injuries to Predict Recovery
- AI-Powered Lung Ultrasound Outperforms Human Experts in Tuberculosis Diagnosis
- AI Identifies Heart Valve Disease from Common Imaging Test
- Novel Imaging Method Enables Early Diagnosis and Treatment Monitoring of Type 2 Diabetes
- Ultrasound-Based Microscopy Technique to Help Diagnose Small Vessel Diseases
- Smart Ultrasound-Activated Immune Cells Destroy Cancer Cells for Extended Periods
- CT-Based Deep Learning-Driven Tool to Enhance Liver Cancer Diagnosis
- AI-Powered Imaging System Improves Lung Cancer Diagnosis
- AI Model Significantly Enhances Low-Dose CT Capabilities
- Ultra-Low Dose CT Aids Pneumonia Diagnosis in Immunocompromised Patients
- AI Reduces CT Lung Cancer Screening Workload by Almost 80%
- Global AI in Medical Diagnostics Market to Be Driven by Demand for Image Recognition in Radiology
- AI-Based Mammography Triage Software Helps Dramatically Improve Interpretation Process
- Artificial Intelligence (AI) Program Accurately Predicts Lung Cancer Risk from CT Images
- Image Management Platform Streamlines Treatment Plans
- AI Technology for Detecting Breast Cancer Receives CE Mark Approval
- Patient-Specific 3D-Printed Phantoms Transform CT Imaging
- Siemens and Sectra Collaborate on Enhancing Radiology Workflows
- Bracco Diagnostics and ColoWatch Partner to Expand Availability CRC Screening Tests Using Virtual Colonoscopy
- Mindray Partners with TeleRay to Streamline Ultrasound Delivery
- Philips and Medtronic Partner on Stroke Care
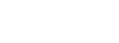
- AI Improves Early Detection of Interval Breast Cancers
- World's Largest Class Single Crystal Diamond Radiation Detector Opens New Possibilities for Diagnostic Imaging
- AI-Powered Imaging Technique Shows Promise in Evaluating Patients for PCI
- Higher Chest X-Ray Usage Catches Lung Cancer Earlier and Improves Survival
- AI-Powered Mammograms Predict Cardiovascular Risk
- Cutting-Edge MRI Technology to Revolutionize Diagnosis of Common Heart Problem
- New MRI Technique Reveals True Heart Age to Prevent Attacks and Strokes
- AI Tool Predicts Relapse of Pediatric Brain Cancer from Brain MRI Scans
- AI Tool Tracks Effectiveness of Multiple Sclerosis Treatments Using Brain MRI Scans
- Ultra-Powerful MRI Scans Enable Life-Changing Surgery in Treatment-Resistant Epileptic Patients
- Novel PET Imaging Approach Offers Never-Before-Seen View of Neuroinflammation
- Novel Radiotracer Identifies Biomarker for Triple-Negative Breast Cancer
- Innovative PET Imaging Technique to Help Diagnose Neurodegeneration
- New Molecular Imaging Test to Improve Lung Cancer Diagnosis
- Novel PET Technique Visualizes Spinal Cord Injuries to Predict Recovery
- AI-Powered Lung Ultrasound Outperforms Human Experts in Tuberculosis Diagnosis
- AI Identifies Heart Valve Disease from Common Imaging Test
- Novel Imaging Method Enables Early Diagnosis and Treatment Monitoring of Type 2 Diabetes
- Ultrasound-Based Microscopy Technique to Help Diagnose Small Vessel Diseases
- Smart Ultrasound-Activated Immune Cells Destroy Cancer Cells for Extended Periods
- CT-Based Deep Learning-Driven Tool to Enhance Liver Cancer Diagnosis
- AI-Powered Imaging System Improves Lung Cancer Diagnosis
- AI Model Significantly Enhances Low-Dose CT Capabilities
- Ultra-Low Dose CT Aids Pneumonia Diagnosis in Immunocompromised Patients
- AI Reduces CT Lung Cancer Screening Workload by Almost 80%
- Global AI in Medical Diagnostics Market to Be Driven by Demand for Image Recognition in Radiology
- AI-Based Mammography Triage Software Helps Dramatically Improve Interpretation Process
- Artificial Intelligence (AI) Program Accurately Predicts Lung Cancer Risk from CT Images
- Image Management Platform Streamlines Treatment Plans
- AI Technology for Detecting Breast Cancer Receives CE Mark Approval
- Patient-Specific 3D-Printed Phantoms Transform CT Imaging
- Siemens and Sectra Collaborate on Enhancing Radiology Workflows
- Bracco Diagnostics and ColoWatch Partner to Expand Availability CRC Screening Tests Using Virtual Colonoscopy
- Mindray Partners with TeleRay to Streamline Ultrasound Delivery
- Philips and Medtronic Partner on Stroke Care