Expo
view channel
view channel
view channel
view channel
view channel
view channel
view channel
RadiographyMRIUltrasound
General/Advanced ImagingImaging ITIndustry News
Events
- Cutting-Edge Technology Enhances Chest X-Ray Classification for Superior Patient Outcomes
- AI Model Accurately Estimates Lung Function Using Chest X-Rays
- High-Powered Motorized Mobile C-Arm Delivers State-Of-The-Art Images for Challenging Procedures
- Injury Prediction Rule Reduces Radiographic Imaging Exposure in Children
- AI Detects More Breast Cancers with Fewer False Positives
- AI Outperforms Clinical Tests at Predicting Alzheimer’s Progress from MRI Scans
- Artificial Intelligence Speeds Up Examination of Heart Images from Magnetic Resonance Imaging
- AI Model Diagnoses Traumatic Brain Injury from MRI Scans With 99% Accuracy
- New MR Image Guidance Software Provides Direct Tumor Visualization during HDR Prostate Procedures
- Brain Connectivity on MRI Predicts Parkinson’s Disease Progression
- PET/CT Imaging Using New Tracing Agent Could Become ‘Gold Standard’ Test for Prostate Cancer Detection
- New Imaging Technique Detects Aggressive Lung and Prostate Cancers
- First Specific PET Scan for TB to Improve Treatment
- PET/CT Superior at Lesion Detection for Head and Neck Paragangliomas than Gold Standard MRI
- New Radiotracer Generates High Quality and Readily Interpretable Images of Cardiac Amyloidosis
- Ultrasound Beam Triggers ‘Nanodroplets' For Targeted Drug Delivery
- Ultrasound Technology Breaks Blood-Brain Barrier for Glioblastoma Treatment
- Implantable Ultrasound Device Could Replace Electrodes for Deep Brain Stimulation
- Robotic Ultrasound Systems to Assist Doctors during Surgery
- Functional Ultrasound Imaging Records Brain Activity through Transparent Skull Implant
- Breakthrough Brain PET System Aids Diagnosis of Neurological Disorders
- Ultra-High-Performance PET System Provides Never Before Seen Brain Images
- Artificial Intelligence Tool Enhances Usability of Medical Images
- New AI Tool Accurately Detects Six Different Cancer Types on Whole-Body PET/CT Scans
- Innovative Imaging Technique Helps Assess Bone Loss after Bariatric Surgery
- Global AI in Medical Diagnostics Market to Be Driven by Demand for Image Recognition in Radiology
- AI-Based Mammography Triage Software Helps Dramatically Improve Interpretation Process
- Artificial Intelligence (AI) Program Accurately Predicts Lung Cancer Risk from CT Images
- Image Management Platform Streamlines Treatment Plans
- AI Technology for Detecting Breast Cancer Receives CE Mark Approval
- GE HealthCare Acquires Intelligent Ultrasound Group’s Clinical Artificial Intelligence Business
- Bayer and Rad AI Collaborate on Expanding Use of Cutting Edge AI Radiology Operational Solutions
- Polish Med-Tech Company BrainScan to Expand Extensively into Foreign Markets
- Hologic Acquires UK-Based Breast Surgical Guidance Company Endomagnetics Ltd.
- Bayer and Google Partner on New AI Product for Radiologists
Expo
view channel
view channel
view channel
view channel
view channel
view channel
view channel
RadiographyMRIUltrasound
General/Advanced ImagingImaging ITIndustry News
Events
Advertise with Us
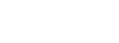
- Cutting-Edge Technology Enhances Chest X-Ray Classification for Superior Patient Outcomes
- AI Model Accurately Estimates Lung Function Using Chest X-Rays
- High-Powered Motorized Mobile C-Arm Delivers State-Of-The-Art Images for Challenging Procedures
- Injury Prediction Rule Reduces Radiographic Imaging Exposure in Children
- AI Detects More Breast Cancers with Fewer False Positives
- AI Outperforms Clinical Tests at Predicting Alzheimer’s Progress from MRI Scans
- Artificial Intelligence Speeds Up Examination of Heart Images from Magnetic Resonance Imaging
- AI Model Diagnoses Traumatic Brain Injury from MRI Scans With 99% Accuracy
- New MR Image Guidance Software Provides Direct Tumor Visualization during HDR Prostate Procedures
- Brain Connectivity on MRI Predicts Parkinson’s Disease Progression
- PET/CT Imaging Using New Tracing Agent Could Become ‘Gold Standard’ Test for Prostate Cancer Detection
- New Imaging Technique Detects Aggressive Lung and Prostate Cancers
- First Specific PET Scan for TB to Improve Treatment
- PET/CT Superior at Lesion Detection for Head and Neck Paragangliomas than Gold Standard MRI
- New Radiotracer Generates High Quality and Readily Interpretable Images of Cardiac Amyloidosis
- Ultrasound Beam Triggers ‘Nanodroplets' For Targeted Drug Delivery
- Ultrasound Technology Breaks Blood-Brain Barrier for Glioblastoma Treatment
- Implantable Ultrasound Device Could Replace Electrodes for Deep Brain Stimulation
- Robotic Ultrasound Systems to Assist Doctors during Surgery
- Functional Ultrasound Imaging Records Brain Activity through Transparent Skull Implant
- Breakthrough Brain PET System Aids Diagnosis of Neurological Disorders
- Ultra-High-Performance PET System Provides Never Before Seen Brain Images
- Artificial Intelligence Tool Enhances Usability of Medical Images
- New AI Tool Accurately Detects Six Different Cancer Types on Whole-Body PET/CT Scans
- Innovative Imaging Technique Helps Assess Bone Loss after Bariatric Surgery
- Global AI in Medical Diagnostics Market to Be Driven by Demand for Image Recognition in Radiology
- AI-Based Mammography Triage Software Helps Dramatically Improve Interpretation Process
- Artificial Intelligence (AI) Program Accurately Predicts Lung Cancer Risk from CT Images
- Image Management Platform Streamlines Treatment Plans
- AI Technology for Detecting Breast Cancer Receives CE Mark Approval
- GE HealthCare Acquires Intelligent Ultrasound Group’s Clinical Artificial Intelligence Business
- Bayer and Rad AI Collaborate on Expanding Use of Cutting Edge AI Radiology Operational Solutions
- Polish Med-Tech Company BrainScan to Expand Extensively into Foreign Markets
- Hologic Acquires UK-Based Breast Surgical Guidance Company Endomagnetics Ltd.
- Bayer and Google Partner on New AI Product for Radiologists